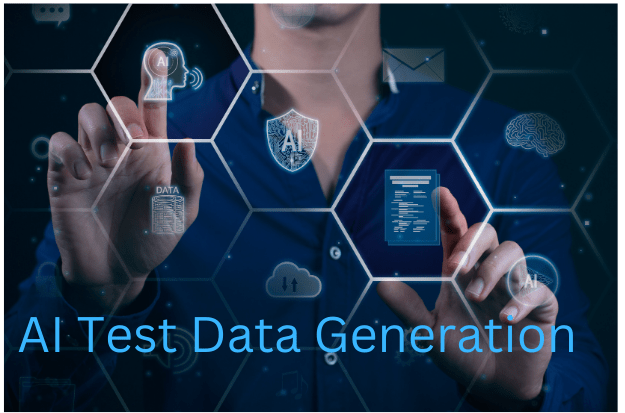
In an age where data is gold, but privacy and accessibility are growing concerns, synthetic data generation has emerged as a game-changer. From enhancing machine learning models to overcoming regulatory roadblocks, synthetic data offers a powerful alternative to traditional data collection. But what exactly is synthetic data, and why is it reshaping the future of data science?
What is Synthetic Data?
Synthetic data is artificially generated information that mimics real-world data. It’s created using algorithms and models, such as generative adversarial networks (GANs), simulation environments, or statistical methods. The goal is to produce data that reflects the patterns, relationships, and properties of actual datasets—without compromising privacy or requiring access to sensitive information.
Why Use Synthetic Data?
-
Privacy and Compliance
Regulations like GDPR and HIPAA make handling real user data increasingly complex. Synthetic data offers a privacy-preserving alternative that allows organizations to train models or test systems without risking user confidentiality. -
Data Scarcity and Cost
In many domains—like autonomous driving or healthcare—gathering high-quality, labeled data is expensive and time-consuming. Synthetic data can fill these gaps, offering virtually unlimited quantities at a fraction of the cost. -
Bias and Fairness Testing
Real datasets often carry human biases. With synthetic data, researchers can create balanced datasets to test how AI models perform across different groups, helping to identify and mitigate bias. -
Scalability and Experimentation
Need to simulate rare edge cases or rapidly test new features? Synthetic data makes it easy to scale up, simulate extreme scenarios, or tailor datasets to specific needs without starting from scratch.
How is Synthetic Data Generated?
There are several techniques for generating synthetic data:
-
Simulation-Based Generation: Used in environments like video games or autonomous vehicle training, this method simulates real-world physics and behavior to generate labeled data.
-
Statistical Modeling: Tools like Bayesian networks or copulas model the relationships between variables and create new instances based on learned distributions.
-
Machine Learning Approaches: GANs and variational autoencoders (VAEs) are popular methods for generating realistic images, text, and even tabular data that closely mirrors original datasets.
Real-World Applications
-
Healthcare: Synthetic patient records allow researchers to innovate without violating HIPAA regulations.
-
Finance: Banks use synthetic transaction data to train fraud detection systems safely.
-
Retail: Companies simulate customer behavior to optimize supply chains and marketing strategies.
-
Autonomous Systems: Self-driving car algorithms are trained on synthetic simulations of roads, pedestrians, and vehicles to prepare for real-world deployment.
Challenges and Considerations
While synthetic data holds promise, it isn’t a silver bullet. Ensuring realism, avoiding hidden biases, and validating against real-world data are all crucial for effective use. Poorly synthetic data generation can lead to flawed models and incorrect insights.
The Future is Synthetic
As AI and machine learning continue to evolve, the demand for diverse, high-quality, and ethical data sources will only grow. Synthetic data stands at the crossroads of innovation and responsibility—enabling progress without compromise. Whether you’re building smarter algorithms or navigating complex regulations, faking it might just be the smartest move of all.