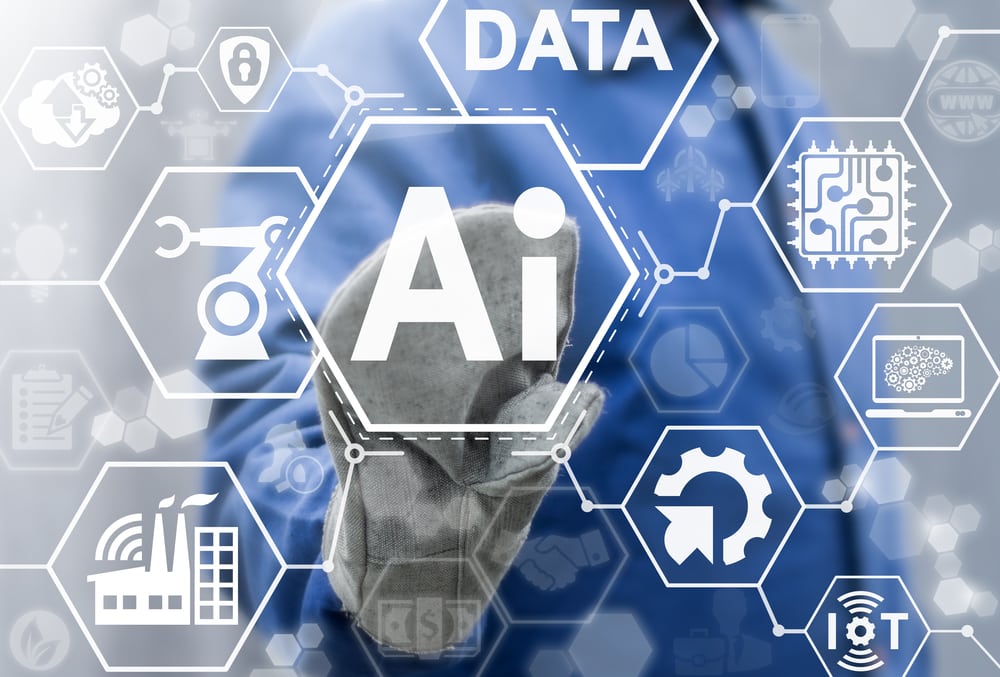
In the age of intelligent automation, data has become the rocket fuel for next-gen marketing strategies. But not just any data—only the right data, fed into the right AI engines, can truly unlock personalized, predictive, and powerful customer experiences. If you’ve ever wondered why some brands seem to know what customers want before they do, the answer lies in a sophisticated blend of machine learning and well-curated data. And with the rise of AI for smarter marketing, knowing which data streams to plug into your algorithms is more important than ever.
According to McKinsey, companies that are data-driven and AI-powered outperform competitors by up to 20% in customer satisfaction and more than 25% in profitability. That means if you’re not already feeding diverse, high-quality data into your marketing AI systems, you’re not just leaving money on the table—you’re probably handing it to your competition.
Here are seven data sources that, when strategically integrated, can significantly enhance your AI marketing capabilities and bring your campaigns to life in ways that were impossible just a few years ago.
1. Customer Behavior Data: The Bedrock of Personalization
One of the most valuable data sources to feed into AI for smarter marketing is customer behavior data. This includes every digital breadcrumb a user leaves behind—clicks, scrolls, product views, purchase history, time spent on pages, and even cart abandonment patterns.
When AI models are trained on this data, they start to understand not just what a customer has done, but what they are likely to do next. For example, if a user often browses winter jackets in October but buys in November, AI can begin recommending cold-weather gear in late September. That’s not just smart marketing—it’s predictive engagement.
This kind of behavioral data can be collected from your website analytics tools, CRM systems, and eCommerce platforms. Feeding this into AI models enables dynamic personalization, such as delivering real-time product recommendations, customizing website interfaces, or timing your emails when users are most likely to engage.
2. Social Media Signals: Tapping into Real-Time Sentiment
Social media platforms are gold mines of unfiltered consumer sentiment, and AI thrives on this kind of real-time, conversational data. From likes and shares to comments, hashtags, and mentions, the content users post or engage with reveals invaluable insights into their interests, pain points, and brand perceptions.
By applying natural language processing (NLP) and sentiment analysis, AI can interpret whether a tweet about your product is positive, negative, or neutral. When integrated into marketing platforms, this allows brands to adjust campaigns on the fly, identify influencers organically, and even detect emerging trends before they hit mainstream.
For instance, a spike in negative sentiment around a new product launch can prompt AI to alert your team, suggest alternative messaging, or even recommend a change in positioning—preventing potential damage in real time.
3. Email Engagement Metrics: Decoding Intent and Readiness
Marketers have long relied on email as a core channel, but traditional metrics like open rates and click-through rates are now being fed into AI to predict user intent and campaign effectiveness. When AI evaluates historical engagement trends—such as how often a user opens your emails, what kind of subject lines resonate, or which links they click—it begins to build a granular understanding of content affinity and buying readiness.
For example, if AI sees that a particular subscriber always clicks on product tutorial links but rarely converts, it may automatically place that user into an educational nurture sequence. Conversely, someone who frequently clicks “Buy Now” links might be tagged as high-intent, triggering personalized offers or retargeting ads.
Integrating this data allows for the automation of segmentation, content customization, and even predictive churn models—all based on how users interact with your emails over time.
4. Customer Support Logs: Discovering Pain Points and Product Gaps
Your customer service logs and chat transcripts are treasure troves of authentic customer feedback. Yet, most companies leave this data untouched. When fed into an AI system, these logs can be analyzed to uncover recurring issues, sentiment trends, or gaps in user experience that might not surface through surveys or reviews.
Imagine an AI engine trained to read thousands of customer service interactions. It can identify that users are frequently complaining about a specific feature being difficult to use or that a particular product has confusing instructions. These insights can be immediately looped back into marketing, informing everything from ad copy to onboarding emails.
Furthermore, this kind of data enhances your ability to craft empathy-driven messaging, because it’s grounded in real frustrations, desires, and experiences voiced by your customers themselves.
5. Search and SEO Data: Revealing Consumer Curiosity
One of the most actionable yet underutilized data sources in AI-powered marketing is search behavior data—both on-site searches and external search engine data. What users type into search bars reflects their current interests, problems, and the language they use to describe them.
By integrating search query data into your AI models, you can surface insights such as which product features are most in demand, what terminology resonates with your audience, or which questions are being asked that your current content doesn’t address. This insight can inform content strategies, PPC campaigns, and even product development.
For SEO professionals, combining AI and search data means identifying long-tail keywords before competitors do, optimizing existing content dynamically, and predicting seasonal demand curves. Tools like Google Search Console, SEMrush, and Ahrefs can feed this data directly into your marketing AI pipelines for smarter forecasting and targeting.
6. Purchase and Transaction Data: Mapping Buyer Journeys
Your sales and transaction history contains patterns that reveal how customers move from consideration to conversion. Feeding this data into AI systems allows you to construct detailed customer journey models, understand purchase cycles, and anticipate repeat buying behavior.
AI can recognize that a customer typically buys a certain product every 90 days and proactively suggest a reorder at just the right time. It can also identify cross-sell or upsell opportunities based on prior purchases. For example, someone who recently bought running shoes might be a good candidate for a fitness tracker or performance apparel.
When this data is linked with behavioral and demographic information, it enables laser-focused lifecycle marketing—driving not just acquisition but also retention and customer lifetime value.
7. Demographic and Psychographic Profiles: Fueling Hyper-Personalization
While behavioral and transactional data tell you what users are doing, demographic and psychographic data helps explain why. Age, gender, income, education, interests, lifestyle preferences, and personality traits can all influence buying behavior, and feeding this data into AI systems enhances the depth and accuracy of audience segmentation.
AI models trained on this data can generate hyper-specific personas and predict how different segments will respond to particular offers, channels, and messages. For example, a 45-year-old tech-savvy professional might be more responsive to LinkedIn ads promoting productivity tools, while a 25-year-old student could respond better to influencer-driven content on Instagram.
Platforms like Facebook, TikTok, and Google Ads already provide granular demographic and interest-based targeting. By aligning this with your internal customer data and feeding it into AI systems, you’re not just reaching people—you’re reaching them in the most contextually relevant way possible.
How to Start Feeding AI the Right Data
While the temptation to integrate everything at once can be strong, the key to success lies in strategic prioritization. Begin with the data you already have access to—whether it’s Google Analytics, CRM exports, or email metrics—and ensure it’s clean, compliant, and structured in a way AI can easily digest. From there, look for gaps in your data strategy that could be filled by third-party tools, APIs, or customer feedback loops.
If you’re serious about turning your marketing team into an AI-driven powerhouse, consider upskilling your team. Enrolling in the best AI marketing course can help you understand data pipelines, model training, and how to choose the right tools for your specific needs. These programs can make the difference between simply collecting data and activating it for tangible results.
Final Thoughts: Turning Data into Your Competitive Edge
Feeding high-quality data into AI systems is not just about automation or efficiency—it’s about amplifying creativity, precision, and customer understanding at scale. With AI for smarter marketing, your ability to listen, learn, and adapt in real time becomes a distinct advantage that static, rule-based systems simply can’t match.
The future of marketing isn’t about guessing what your audience wants. It’s about knowing—because the data already told you. And the AI? It made sure you listened.